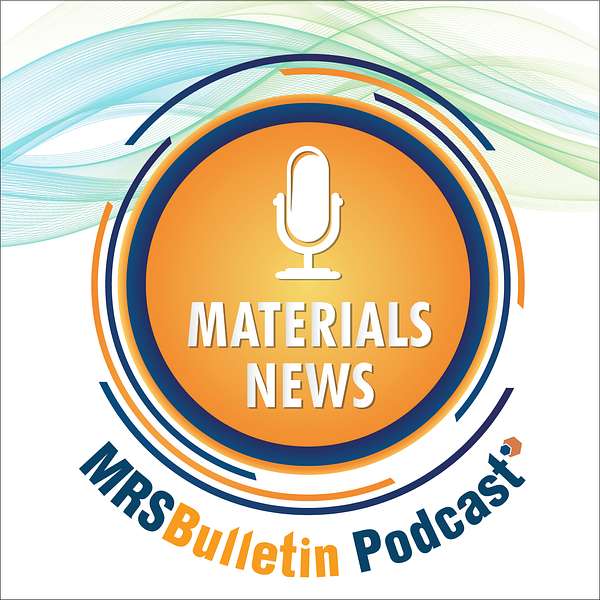
MRS Bulletin Materials News Podcast
MRS Bulletin Materials News Podcast
Episode 14: Thin film patterns classified by machine learning
Sophia Chen of MRS Bulletin interviews Alex Hexemer of Lawrence Berkeley National Laboratory in California, and Daniela Ushizima and Shuai Liu of the University of California, Berkeley about their design of multiple Convolutional Neural Networks (CNN) to classify nanoparticle orientation in a thin film by learning scattering patterns. Read the article in MRS Communications.
Transcript
SOPHIA CHEN: Materials researchers come from around the world to study their samples in the beamline at the Advanced Light Source facility, located at Lawrence Berkeley National Laboratory in California. Alex Hexemer, a senior scientist at the facility, tells me that they’re currently upgrading the machine, so that it can take much more data, much more quickly.
HEXEMER: The amount of data you’re going to create is so large that A, you can’t take it home on a hard drive anymore, nor can you start looking at the data anymore. It’s just too big. Some of the detectors here are going to run at thousands of frames a second. It becomes unmanageable from a human point, so we have to transition to more automated approaches.
CHEN: So Hexemer and his collaborators decided to try a machine learning approach to quickly classify and process x-ray images. To develop their image classification algorithm, they worked with frequency-space pictures of thin films made of polymers, about 100 nm thick. Scientists image these thin films at the facility. They consist of intricate geometrical patterns on the nanometer scale, which researchers try to engineer to create specific materials properties. For example, Hexemer explains that one future application is a printable solar panel. In the future, people might be able to print photovoltaics made of thin film polymers. But first, they need to figure out what nanometer structures work the best.
HEXEMER: To try to understand the efficiency of the material, we have to understand the morphology.
CHEN: They came up with seven different categories of thin film patterns. One of Hexemer’s computer science collaborators, Dani Ushizima, explains that they had to show the computer millions of examples.
DANIELA USHIZIMA: This neural network base will build a mathematical model that will represent the different patterns.
CHEN: They found they could classify images successfully into the seven categories 94% of the time.
USHIZIMA: The training process might take a long time—hours. But the feedback, to classify a scattering pattern, this is coming on the millisecond.
CHEN: The images they classified were simulations of thin films rather than real data.
HEXEMER: We want to have better and better simulations close to real and partially disordered systems. And that is very difficult.
CHEN: The team brought together experts from materials science and computer science. Shuai Liu, a member of the team, says to expect more collaborations between the disciplines.
SHUAI LIU: We point out a very important direction in future research is to combine machine learning, which has been well developed in recent years, with a lot of characterization techniques.
CHEN: My name is Sophia Chen from the Materials Research Society. For more news, log onto the MRS Bulletin website at mrsbulletin.org and follow us on twitter, @MRSBulletin. Thank you for listening.