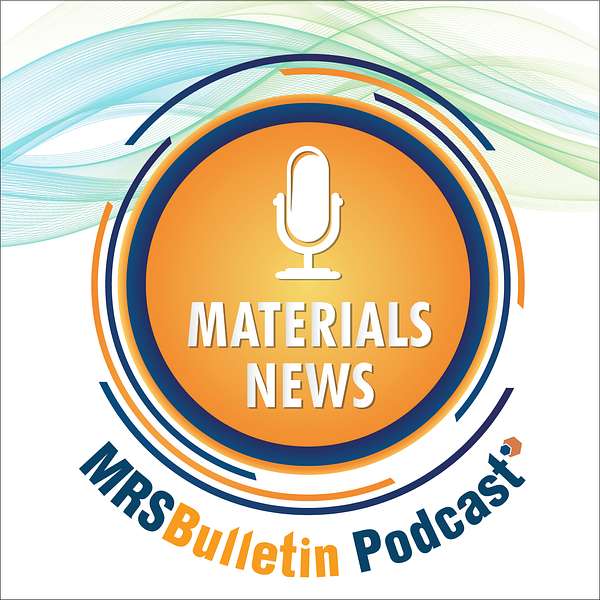
MRS Bulletin Materials News Podcast
MRS Bulletin Materials News Podcast
Episode 1: Strain engineering guided by machine learning
Omar Fabián of MRS Bulletin interviews Ju Li of Massachusetts Institute of Technology about applying machine learning to elastic strain engineering of semiconductor materials at the nanoscale. The research team presents a framework for guiding strain engineering whereby materials properties and performance could be designed. Read the article in Proceedings of the National Academy of Sciences.
Transcript
OMAR FABIÁN: There are innumerable ways to alter a material’s properties. For crystalline materials it comes down to lattice structure, to the shape of the unit cell. That’s the key to unlocking new macroscopic properties from the same old material.
JU LI: We think we know silicon…But what is interesting is if you put 5% tension on silicon or 5% shear that becomes a different material.
OF: That’s Prof. Ju Li, a professor of nuclear science and engineering at MIT, talking about elastic strain engineering.
JL: This is not, you know, you take a piece of metal and you can bend it 30, 50%. Those are plastic strain. The amount of elastic strain that a conventional metal can sustain is no more than 0.2, 0.3%. But with nanomaterials, we can talk about more than 1% elastic strain. And not just near defects or near some special locations, but throughout the entire component that you are using.
OF: Going nano opens up new degrees of freedom for accessing bandgaps that are otherwise off limits. One percent strain, for example, is enough to bump silicon’s bandgap such that the material’s electron mobility jumps by more than 50%. But at about 15% strain, you’re able to wipe out silicon’s bandgap altogether—transforming it into a metal. Prof. Li’s team is using neural networks to learn the quickest, lowest-energy pathways to get from a set of strain conditions to different bandgap energies in materials like silicon and diamond.
JL: It turns out that if you want to make silicon a metal, then the strain path you impose should not be a straight line. So perturbation theory doesn’t work. The best way to make silicon in the least amount of energy to reduce its bandgap is actually a pretty curved path. And so that’s sort of the power of ML. It’s a fast-acting model, it can do all kinds of projections or visualizations that you simply cannot do on a point-by-point calculation. You can also ask other questions, like how do I improve its thermoelectric figure of merit? Or what’s the fastest way to make it have this kind of optical signature? This is kind of like Alice in Wonderland. There is suddenly a big space that opens up and that can be a little bewildering at the beginning because we are sort of looking at each other and saying what is the first device we’re going to design. It’s quite a big space, and we feel that just on our own group, our own power, we probably cannot explore it all. So we’d really like the community to jump in and help in this effort of strain engineering because it’s going to have long-term consequence on human civilization—just as much as chemical metallurgy has.