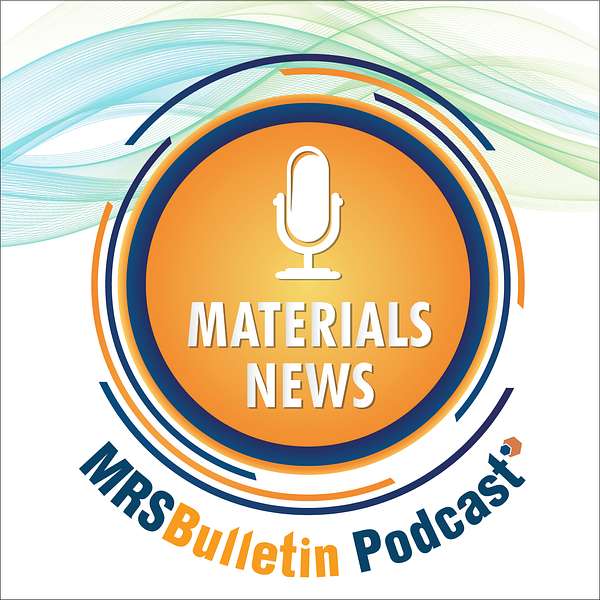
MRS Bulletin Materials News Podcast
MRS Bulletin Materials News Podcast
Episode 4: Meet research team member SARA
In this podcast episode, MRS Bulletin’s Sophia Chen interviews Carla Gomes, Michael Thompson, and Max Amsler of Cornell University about their robot, SARA—Scientific Autonomous Reasoning Agent. Unlike commonly known artificial intelligence (AI) applications such as the neural networks that enable image recognition, SARA performs within a closed loop system through a type of AI known as active learning, which allows the system to reason without a lot of training data. Within 30 minutes, SARA figured out how to make delta phase bismuth oxide and cool it to room temperature, saving the research team two full days of experiments.
SOPHIA CHEN: Welcome to MRS Bulletin’s Materials News Podcast, providing breakthrough news & interviews with researchers on the hot topics of biomaterials, quantum materials, sustainability, artificial intelligence, perovskites, and robotics. My name is Sophia Chen. Carla Gomes of Cornell University, introduces me to a member of her group named SARA. Like other members of her team, SARA works on discovering new materials to enable technologies for sustainability, such as fuel cells. But SARA differs from the rest of the team in one key aspect: SARA is a robot.
CARLA GOMES: SARA stands for Scientific Autonomous Reasoning Agent.
SC: SARA lives in the cloud as an artificial intelligence-trained system that controls machines in the laboratory. The team used SARA to study a material called bismuth oxide. Specifically, they had SARA learn to synthesize a new phase of bismuth oxide, known as the delta phase. This material is very good at transporting oxygen ions, which means it could benefit electrochemical fuel cells, as team member Michael Thompson says.
MICHAEL THOMPSON: It is the best of the ionic conductors, solid-state ionic conductors that are currently known.
SC: However, the delta phase wasn’t a practical material. It’s synthesized at high temperatures, and previously, people didn’t know how to make it and cool it to room temperature without degrading. So SARA synthesized bismuth oxide under varying conditions, took optical measurements of those samples, and analyzed those measurements. Over many iterations, SARA figured out how to make delta phase bismuth oxide and cool it to room temperature, with a technique called laser spike annealing. And it did it all much faster than possible with human scientists, says team member Max Amsler, a computational materials scientist at Cornell.
MAX AMSLER: If you did everything exhaustively, it would take about two full days to do the whole experiment. But we can do it with SARA within 30 minutes, roughly.
SC: SARA is what’s known as a closed loop system. This means that SARA can synthesize a material, take measurements of that material, analyze those measurements, and then figure out how to update the material recipe to get closer to a desired material property. Then it synthesizes that material, and the cycle continues, all without human intervention. The closed loop is what sets SARA apart from other AI-driven materials discovery systems. SARA learned to do all this via a type of AI known as active learning, which allows the system to reason without a lot of training data. This is in contrast with AI applications most familiar to the general public, such as the neural networks that enable image recognition, which require massive amounts of labeled training data to work.
CG: For SARA to make decisions, it incorporates information about materials science, and in particular, about, for example, the characteristics of the laser spike annealing.
SC: SARA’s capability extends beyond bismuth oxide. The team is already using SARA for other materials discoveries. The vision is for Sara to serve as a robot scientist that works alongside the rest of the team.
CG: SARA will encapsulate the scientific method, so, you know, from formulating hypotheses, the designing and conceiving experiments, running the experiments, interpreting experiments and generating new knowledge and new hypotheses.
SC: In doing so, SARA frees up time for researchers to spend more time on higher-level scientific questions rather than the tedious work of materials synthesis and characterization. Amsler says that they’d like to add more complex and different capabilities to SARA, for example, adding the ability to take more types of measurements to inform the synthesis process.
MA: The way the system was designed is that we can keep adding more agents to it and you know, make the SARA infrastructure more and more complex.
SC: The team has also published the AI software behind SARA as open-source code. This work was published in a recent issue of Science Advances (10.1126/sciadv.abg4930). My name is Sophia Chen from the Materials Research Society. For more news, log onto the MRS Bulletin website at mrsbulletin.org and follow us on twitter, @MRSBulletin. Don’t miss the next episode of MRS Bulletin Materials News – subscribe now. Thank you for listening.